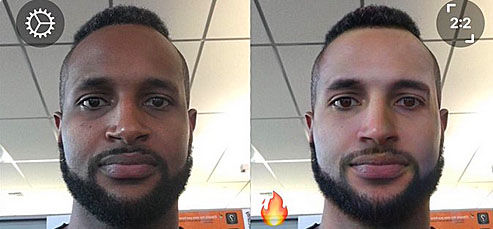
It’s not just us; many of our Artificial Intelligence systems are racist too. This is particularly true in cybersecurity and law enforcement, sometimes with dangerous consequences for people of color.
AI isn't inherently race biased. It learned it from us. Now is the perfect time to fix it.
Face recognition has become the poster child for this problem, with police departments using it to identify and arrest protesters after the death of George Floyd. This sparked a public backlash, with Amazon, Google, and Microsoft promising to stop selling face recognition tools to police. We soon learned that Amazon’s change was temporary (a one-year “moratorium”) and Microsoft’s was selective (they still sell face recognition to federal agencies like DEA).
Face recognition systems tend to have higher failure rates on black faces than white faces. This can have serious consequences. A false positive, where the system thinks photos of Al and Bob are the same person, can lead to Bob’s arrest for Al’s crimes. A false negative, where the system thinks two photos of Al are different people, can lead to Al evading arrest.
Race bias isn’t limited to face recognition systems. It pervades many Artificial Intelligence (AI) applications, especially those that rely on artificial neural networks. These systems are trained on large data sets to learn a task. If the training data contains an inadvertent bias, such as an insufficient number of black faces, then the system can acquire biased behavior.
Examples of racist AI run from silly to sinister:
If you Google “cute baby” all the results show white babies.
Some restroom soap dispensers activate for white hands but not black hands.
Speech recognition used in personal assistants (Alexa, Siri, etc.) have trouble understanding African-American Vernacular English.
Medical risk rating tools can steer black people away from heart surgery, kidney transplants, breast biopsies at higher rates than whites.
Criminal risk rating tools rate blacks higher than whites as risk to reoffend, which is one reason why African-Americans get longer sentences and higher rates of imprisonment.
Some self-driving cars are more likely to drive into black people.
As America undergoes a reckoning over racial injustice, those of us who work in technology must do our part, striving to keep bias out of systems that society increasingly depends on.
The primary ways bias creeps into AI applications are training and testing:
Training: Data sets used to train neural networks must be diverse. For example, a collection of images used to train a face recognition system must include enough black faces to allow the system to achieve proficiency in black face recognition.
Testing: AI tools must be tested against diverse subjects. For example, a face recognition system should achieve comparable false match / mismatch rates for black faces as for white faces.
Those of us who evaluate or purchase AI-driven systems should insist on this. Black data matters. Demand demographic test results from vendors that show a system’s performance across the spectrum of race, ethnicity, gender, etc.
Michael McCormick is an information security consultant, researcher, and founder of Taproot Security.
Comments